
February 6 • 10 min read
Power and Prediction: The Disruptive Economics of Artificial Intelligence - What's new, why it matters and some food for thought
In essence, what is the disruptive nature of AI? Why it has been taking so long for the new AI systems to be widespread? What are the key challenges and success factors for building AI systems?
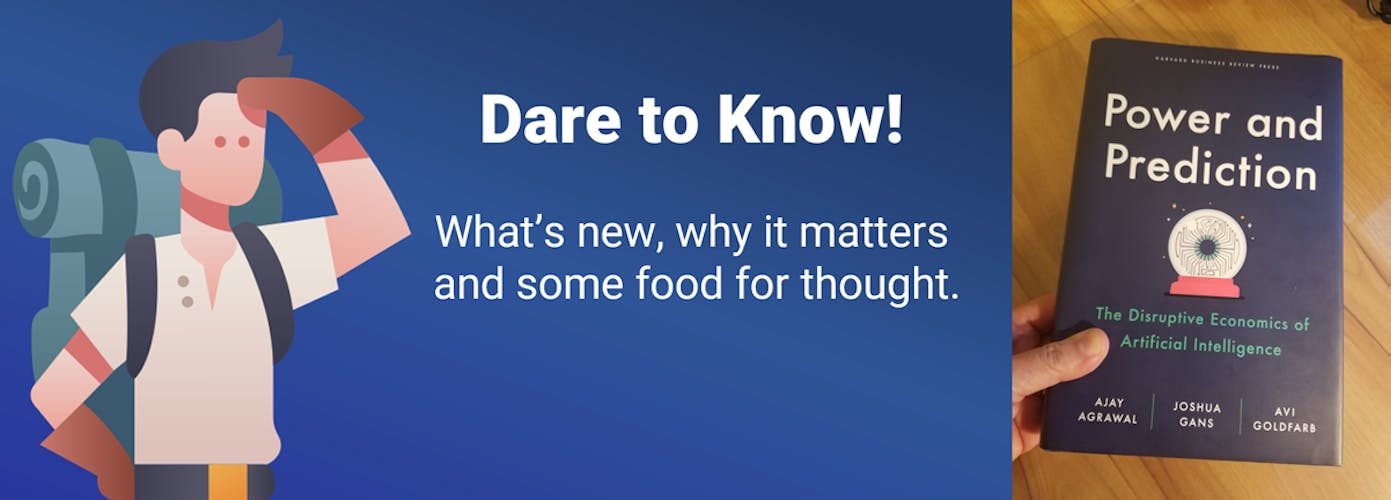
What's new
Power and Prediction: The Disruptive Economics of Artificial Intelligence, by Ajay Agrawal, Joshua Gans, and Avi Goldfarb is an exciting book written by economists with a fresh perspective on the disruptive power of AI. It was timely published in November 2022, a couple of months before ChatGPT astonished the world with interactive, coherent, and context-aware conversations with human beings, achieving 100M users in its first two months.
In their first book, Prediction Machines, the authors explained the economic properties of AI. In this one, they focus on the economics of building new systems based on AI. The authors use the general adoption process of electricity, a disruptive technology that changed the world, as a framework to uncover critical aspects of the challenges we’ve been facing in designing and adopting new AI systems.
The book is organized into six parts that gradually evolve and build on top of each other using simple language and concepts firmly grounded in the foundations of economics. Readers with a background in technology like me may find the narrative sometimes convoluted; however, it makes sense to reiterate basic concepts as necessary to ensure a solid ground for the final and concluding parts of the book.
From my perspective, the authors articulate throughout the book insights on the following vital questions:
In essence, what is the disruptive nature of AI?
Decisions are riddled in everything we do and have two vital separate components: prediction and judgment. As human beings, we’re constantly assessing the prediction (probabilities of events) and judgments (rewards from the consequences of those potential events) regardless of whether we decide.
In essence, AI provides prediction, a way of managing uncertainty, and creating value by improving decision-making. It’s disruptive because it forcefully decouples prediction from judgment, potentially providing better predictions (higher accuracy) at lower costs.
AI can also unlock new decisions, creating a ripple effect of changes that are hidden from view. These new decisions challenge existing rules and standard operating procedures previously put in place to hide uncertainty and simplify decisions (like leaving home for the airport 2.5 hours before flight departure time). The problem with challenging existing rules is that they admit errors and tolerate mistakes, and their replacement requires further redesign considerations that might not be worthwhile unless a system’s mindset is accepted and adopted.
Consider, for example, the decisions about buying ingredients for a restaurant at a local food market. If too much is purchased, it will become a waste. If too little is purchased, customers will be dissatisfied with one or more unavailable menu items, and revenue will be lost. Improving predictions based on the demand can substantially improve customer satisfaction and restaurant profitability. If generally adopted by most of the local restaurants, the improved prediction system will impact food distributors that service that market, causing fluctuations in the orders received and requiring them to challenge their existing sourcing rules (the “AI Bullwhip,” according to the authors). Further propagating better predictions through this value chain might disrupt the whole food production and distribution network.
As economists studying the new developments in AI over the last decade, we have come to see our role as one of cutting through the hype.
"Power and Prediction: The Disruptive Economics of Artificial Intelligence", by Ajay Agrawal, Joshua Gans, and Avi Goldfarb
Ultimately AI does not provide insights, it provides predictions that decoupled from judgment might be disruptive.
Why it has been taking so long for the new AI systems to be widespread?
Using the adoption of electricity in the US as a reference pattern, the authors argue that realizing a technology’s promise doesn’t happen immediately after demonstrating its capability. It took approximately 40 years for half of US households to have electricity since Thomas Edison invented the light bulb. Those were “The Between Times” of electricity adoption.
While the future of AI is uncertain, the path to significant productivity gains relies on understanding what it can offer. Like in the electricity adoption process, AI enables three categories of solutions:
- Point solutions that improve isolated parts of a system, providing local efficiencies. In the early days of electricity, entrepreneurs focused on replacing steam engines with electric power generators to reduce power bills. There was no impact on the layout and infrastructure of factories.
- Application solutions improve interdependent components or portions of a system, redesigning them to provide significant efficiencies. After the replacement of steam engines, entrepreneurs explored opportunities to install electric motors at each machine in a group of machines, only paying for power when machines were being used.
- System solutions improve the way something is done in its entirety, maximizing overall efficiency. Electricity provided the flexibility entrepreneurs like Henry Ford required to optimize the overall factory layout and design by organizing and spreading production assets and workers in a line instead of concentrating them around steam engines.
The authors argue that AI enables system-level innovations by decoupling prediction from judgment. When “decisions are driven not by who does prediction and judgment together but who is best to provide judgment using AI prediction.” These system solutions are hard to realize since they reshape industries, shift power, require organizational changes, and face significant resistance.
Cars were able to be better than horses, but cars needed gas stations, good roads, a whole set of new laws.
"Power and Prediction: The Disruptive Economics of Artificial Intelligence", by Ajay Agrawal, Joshua Gans, and Avi Goldfarb
What are the key challenges and success factors for building AI systems?
Using examples from various industries like healthcare, public health, insurance, transportation, search engines, etc., the authors discuss the challenges of adopting AI applications and system solutions.
AI point solutions don’t require any restructuring; they are just new features for the existing systems, and the return on investment (ROI) comes from localized cost reductions based on improved predictions. As redesign and restructuring opportunities are uncovered by decoupling prediction from judgment, mechanisms put in place to manage uncertainty need to change, and the people and organizations that benefit from them might not accept any changes. For example, the authors mention that the lead times to get to airports are driven by the uncertainty of traffic and security lines. Modern airport shopping centers benefit from that uncertainty. They might oppose any AI application solution that helps travelers to manage uncertainty better and leave their homes just in time to board their flights.
If you never missed the plane, you’re spending too much time in airports
Economist George Stigler
Machines don’t have power; they can only provide improved predictions. Humans make judgments, sometimes embedded in algorithms, and are the ones who ultimately make decisions. When AI solutions challenge the status quo, some stakeholders might resist change. It can be exemplified by the resistance posed by local politicians from Flint, Michigan, to the AI solution capable of predicting which pipes likely contain lead much more accurately than any other person or mechanism. Ultimately, the court decided that the AI system attended to the greater good and should prevail, cementing new decision rights.
When it’s challenging to contemplate new system design opportunities, the authors propose a method and a tool to build an AI solution on a blank slate (“The AI Systems Discovery Canvas”). It helps with the identification of the minimum essential decisions required to be made by an industry, considering high-fidelity prediction machines are available. It also helps to evaluate how adopting AI prediction may lead to disruption and how to consider whether a system-level innovation is required.
As critical success factors, there’s a whole chapter about them (“Accumulating Power”) and other chapter sections that discuss them. In a nutshell, the authors mention the following:
- Better and cheaper algorithms and data: both are required in tandem; however, AI is different from other technologies because it learns. The more it’s used, the better. Early adoption of AI is not necessarily a moat if the prediction does not improve with time. High-quality data with breakthroughs in continuous algorithmic learning can displace first-movers.
- Minimum viable predictions: first-mover advantages depend on how good the prediction needs to be to enter the market. Since software is not a capital-intensive business, the critical success factor is data, not only training data but feedback data capable of improving predictions and making it harder for others to compete. The advantage isn’t launching first, it’s launching with collecting feedback data.
- Fast feedback loops: improving prediction with feedback data creates a race between competitors and amplifies “Sutton’s endogenous costs,” where it might be impossible to catch up to a competitor that falls behind. Being early with a fast feedback loop might be a considerable advantage.
- Differentiated prediction: some AIs appeal to different groups or businesses, so domain knowledge is vital. Many AIs are only differentiated by quality (a term that requires a precise definition depending on the use case).
Interestingly, the authors move many steps forward beyond the lack of transparency and system bias public outcries of AI systems. In a chapter dedicated to this topic (epilogue), they build the case for leveraging AI systems to detect and fix discrimination in human and machine predictions. They argue that measuring discrimination by machine is straightforward while doing the same with humans is hard.
Changing algorithms is easier than changing people: software on computers can be updated; the ‘wetware’ in our brains has far proven much less pliable.
Sendhil Mullainathan, “Biased Algorithms Are Easier to fix Than Biased People.” New York Times, December 2019.
Why it matters
Recent advances in Generative AI, precisely in Large Language Models (LLMs), have been revolutionizing the field of natural language processing and taking the “AI Hype” to an extreme. LLMs have the potential to become a backbone that supports the growth and development of numerous industries. Their versatility and adaptability make them powerful tools for innovation and problem-solving. At the same time, there is growing unease around whether the behavior of these systems can be rendered transparent, explainable, unbiased, and accountable.
As plain citizens, we need to know how our lives are changing with the widespread usage of AI, develop our own opinions, and have meaningful discussions among ourselves, our leaders, lawmakers, and policymakers on maximizing its benefits and mitigating associated risks.
Books like this one, written by subject matter experts outside the technology domain, enrich the conversation and stimulate pondering in a general audience that wants a common-sense explanation of what they are, how they work, and how they can (and already are) impact their lives.
This point of view from economists and university professors heavily engaged in nurturing entrepreneurship deconstructs the technology hype into its “boring” components and exposes its business-related properties.
Food for thought
Finding reference patterns capable of articulating the impact and underlying adoption processes of disruptive technologies is reassuring. I doubt we've yet realized the potential and the essence of AI, which I prefer, for various reasons, to call what we have today "machine learning."
We also don't know how long "The Between Times" will take for this technology; however, we desperately need new mindsets, policies, laws, and regulations to deal with it. Deepfakes, fake news, and biased systems abound.
As the authors say, "the future of AI is uncertain" but it is already impacting our lives.